Historical financial data provides the foundation for precise earnings forecasting and comparative financial analysis. Financial teams can track trends, benchmark progress, and make more accurate risk assessments by analyzing past performance. Historical insights are particularly valuable in earnings models, revealing seasonal patterns and long-term growth indicators that shape strategic decisions.
AI-driven tools simplify the extraction and analysis of historical financial data, enhancing speed and accuracy while reducing manual errors.
Key Takeaways
- Historical data analysis supports accurate financial decision-making and risk management.
- Using past trends allows businesses to identify new opportunities and refine forecasts.
- Leveraging historical insights enhances resilience and keeps businesses ahead.
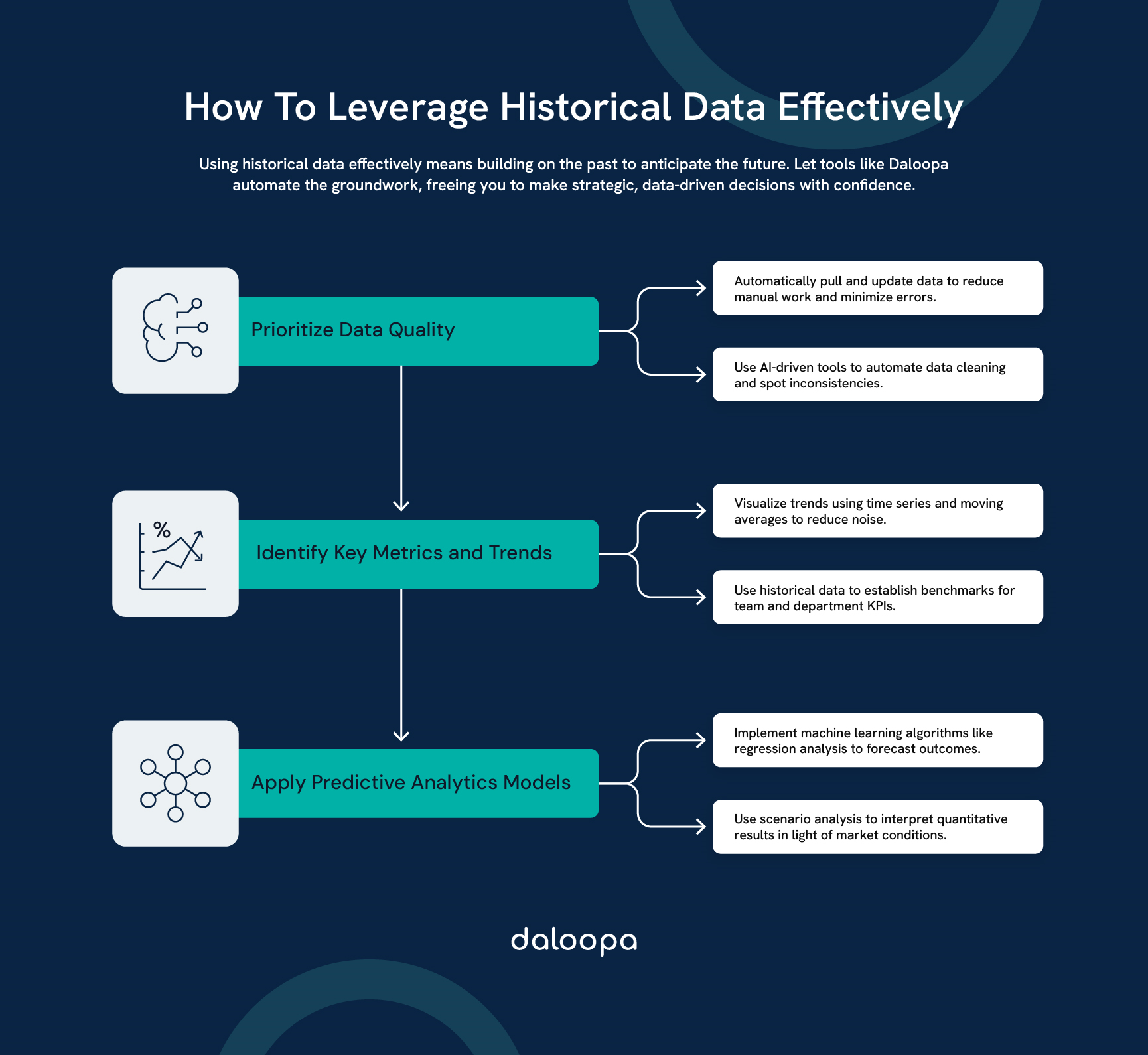
Historical Financial Data’s Impact on Decision-Making
Historical financial data helps identify trends, patterns, and correlations that inform strategies. Analyzing past events and outcomes provides insights that refine choices and forecasts.
Data science techniques extract actionable insights from historical datasets. Statistical models and machine learning algorithms reveal hidden connections and anticipate scenarios, enhancing financial decision-making accuracy.
Combining quantitative assessments with qualitative judgment provides the necessary context, ensuring data analysis captures the broader picture beyond what raw figures reveal.
Core benefits of historical data analysis:
- Enhanced risk evaluation
- More precise forecasting
- Recognition of seasonal trends
- Improved resource planning
Historical data supports learning from past mistakes and enables organizations to build on achievements and avoid past errors, refining processes over time for reliable results.
The Importance of Historical Financial Data in Business and Investment Forecasting
Historical data forms the backbone of sound business and investment forecasting, offering essential insights into past economic trends and market behaviors that guide future planning.
Challenges in Utilizing Historical Data
Historical data presents several challenges in financial modeling, such as ensuring data consistency across diverse sources. Financial models often integrate data from multiple systems, and discrepancies or inconsistencies can lead to inaccurate insights. Another challenge involves the complexity of integrating real-time financial feeds into models.
AI can mitigate these challenges by automating data normalization and continuously updating financial data models with the latest financial feeds, ensuring consistency and accuracy without the manual workload.
Daloopa’s AI-driven tools, for instance, streamline this process by seamlessly integrating real-time data, correcting inconsistencies, and providing up-to-date financial data models. These capabilities enable finance professionals to focus on deeper analysis and financial decision-making while AI handles the heavy lifting of data management.
Best Practices for Leveraging Historical Data
Effectively using historical data in finance requires best practices that ensure data accuracy, relevance, and model adaptability. Leveraging AI to parse and clean data enhances accuracy, addressing issues like inconsistent or incomplete information. Research from institutions like the CFA Institute highlights how AI-driven tools reduce manual errors and improve data consistency, allowing analysts to focus on strategic insights.
Ensuring Data Integrity and Consistency
Data quality is paramount for accurate analysis. One key practice is to employ automated data-cleaning processes, which detect and correct inconsistencies across large datasets, including handling missing values, correcting outliers, and standardizing formats. Data normalization, for instance, aligns historical data from diverse sources, making it easier to conduct accurate comparative analysis.
Quantitative and Qualitative Methods
Quantitative approaches such as statistical analysis, regression, and machine learning models uncover patterns and forecast outcomes. Techniques like time series analysis identify cyclical trends, while smoothing methods, such as moving averages, clarify long-term patterns.
On the qualitative side, scenario analysis offers context and depth. Combining quantitative precision with qualitative insights creates a well-rounded understanding of financial trends.
Trend Analysis and Predictive Analytics
Trend analysis visualizes patterns in historical data through tools like line charts and scatter plots, making complex data more accessible. Predictive analytics then takes trend analysis a step further, using machine learning models such as neural networks to forecast future outcomes. To ensure model accuracy, metrics like root mean squared error (RMSE) and mean absolute error (MAE) are used, with validation against separate data sets to prevent overfitting.
Integrating AI-powered tools simplifies the data preparation process and supports accurate forecasting. By automating tasks like data normalization and real-time updates, these tools allow finance professionals to enhance their financial decision-making processes, maximizing their models’ strategic value.
Case Studies and Real-World Applications
The value of historical data shines through in practical examples where it has driven business success and helped avoid pitfalls.
Successful Use of Historical Financial Data in Business Strategy
Amazon’s recommendation system, grounded in historical purchase data, generates up to 35% of its revenue. Tailoring product suggestions based on past customer behavior enhances user experience and drives sales.
Walmart optimized inventory by pairing historical sales data with weather insights, cutting out-of-stock issues, and boosting customer satisfaction.
Lessons Learned from Past Mistakes
Target’s 2013 data breach exposed the importance of strong data security. Impacting 70 million customers, it cost $18.5 million in settlements and harmed trust, underlining the necessity for robust data protection measures.
Building a Resilient and Data-Driven Future
A new era in financial decision-making emerges as historical data combines with modern analytics to create resilient organizations, enhancing planning and resource management.
AI and data tools process large datasets rapidly, revealing patterns that traditional methods might miss. Such advancements enable proactive planning, especially for disaster and climate challenges, by studying past events and preparing for future crises.
This data-centric approach benefits resource allocation by allowing:
- Prioritization of infrastructure upgrades
- Optimization of emergency responses
- Enhanced urban planning
Integrating historical data with current analysis forms a comprehensive decision-support system. Leaders can draw from past experiences while factoring in present-day insights.
Cities evolve into more adaptive environments through these strategies. Learning from historical precedents and community contexts strengthens their capacity to face new challenges.
In this future vision, data shapes every significant decision, merging experience with innovation to build a path of stability and progress.
Let Daloopa Make Historical Financial Data Work for You
With Daloopa’s AI-driven automation, you can harness historical financial data without the hassle of manually searching each time. By automating data extraction and analysis, Daloopa empowers financial teams to access consistent, up-to-date information effortlessly, allowing you to focus on strategic insights that drive growth. Check out available plans or request a demo.